What to do about algorithmic decision-making
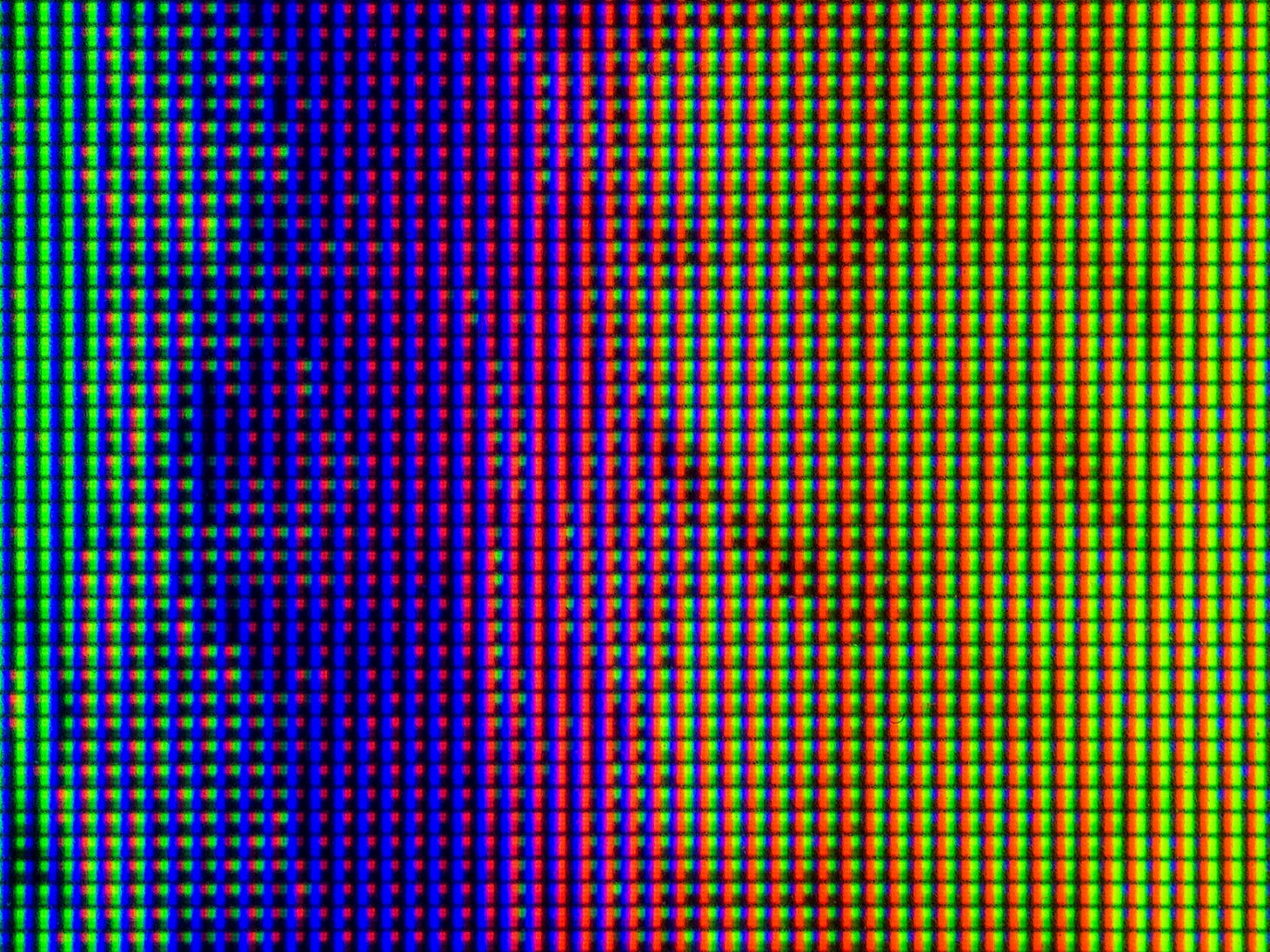
To take advantage of the opportunities algorithmic decision-making (ADM) offers in the area of participation, one overall goal must be set when ADM processes are planned, designed and implemented: ensuring that participation actually increases. If this is not the case, the use of these tools could in fact lead to greater social inequality.
In sum, the opportunities and risks in the examples presented here point to a number of general factors related to ADM processes that can critically affect participation. These factors involve different aspects of the overall socio-informatic process and can be found on different levels. Here are three examples:
- Shaping ADM processes on the micro and macro level: Choosing data and setting criteria at the startof a development process can themselves reflect normative principles which sometimes touch on fundamental social issues.
- Structure of suppliers and operators on the macro level: Having a range of ADM processes andoperators can increase participation (e.g. through credit assessments of people who have not been partof the system in the past), can make it easier to avoid the ADM process and can expand possibilities forfalsification. Conversely, monopolistic structures increase the risk that individuals will “fall out of the system” and get left behind.
- Use of ADM forecasts on the micro, meso and macro level: The interplay of technology, society andindividuals has a major impact on how and when algorithms are used and the influence they thus have.Key questions that must therefore be asked are: How do people (ADM developers and users, and thegeneral public) deal with automated predictions? Do the processes include the possibility of challengingADM results?
What are needed here are additional systematic analyses of the potential shortcomings of ADM processes on different levels — from the definition of the goals and the efforts to measure the issues at hand, to data collection, the selecting of algorithms and the embedding of processes in the relevant social context. Criteria are needed for determining the benefits of ADM processes on all levels and in all steps. The responses discussed here can provide initial impetus for addressing these issues:
1. Ensure falsifiability
ADM processes can learn asymmetrically from mistakes. “Asymmetric” means that the system, by virtue of the design of the overall process, can only recognize in retrospect certain types of its own predictions which proved incorrect. When algorithms learn asymmetrically, the danger always ex-ists that self-reinforcing feedback loops will occur.
Example: Recidivism predictions used in the legal system
2. Ensure proper use
Institutional logic can lead to ADM processes being used for completely different purposes than originally envisioned by their developers. Such inappropriate uses must be avoided.
Example: Predicting individual criminal behavior
3. Identify appropriate logic model for social impact
Algorithm-driven efficiency gains in individual process steps can obscure the question of whether the means used to solve a social problem are generally appropriate.
Example: Predicting lead poisoning
4. Make concepts properly measurable
Social phenomena or issues such as poverty and social ine-quality are often hard to operationalize. Robust benchmarks developed through public discussion are therefore helpful.
Example: Predicting patterns of poverty
5. Ensure comprehensive evaluation
The normative power of what is technically feasible all too easily eclipses the discussion of what makes sense from a social point of view. For example, the scalability of machine-based decisions can quickly lead to situations in which the appropriateness and consequences for society of using ADM processes have neither been debated nor verified.
Example: Automatic face-recognition systems
6. Ensure diversity of ADM processes
Once developed, the decision-making logic behind an ADM process can be applied in a great number of instances with-out any substantial increase in cost. One result is that a limited number of ADM processes can predominate in certain areas of application. The more extensive the reach, the more difficult it is for individuals to escape the process or its conse-quences.
Example: Preselection of candidates using online personality tests
7. Facilitate verifiability
Frequently, no effort is made to determine if an ADM process is sufficiently fair. Doing so is even impossible if the logic and nature of an algorithm is kept secret. Without verification by independent third parties, no informed debate on the opportu-nities and risks of a specific ADM process can take place.
Example: University admissions in France
8. Consider social interdependencies
Even when use is very limited, the interdependences between ADM processes and their environment are highly complex. Only an analysis of the entire socio-informatic process can reveal the relationship between opportunities and risks.
Example: Location-specific pre-dictions of criminal behavior
9. Prevent misuse
Easily accessible predictions such as scoring results can be used for inappropriate purposes. Such misuse must be pre-vented at all costs.
Example: Credit scoring in the US
This is an excerpt from the working paper “Wenn Maschinen Menschen bewerten – Internationale Fallbeispiele für Prozesse algorithmischer Entscheidungsfindung”, written by Konrad Lischka and Anita Klingel, published by the Bertelsmann Stiftung under CC BY-SA 3.0 DE.
This publication documents the preliminary results of our investigation of the topic. We are publishing it as a working paper to contribute to this rapidly developing field in a way that others can build on.